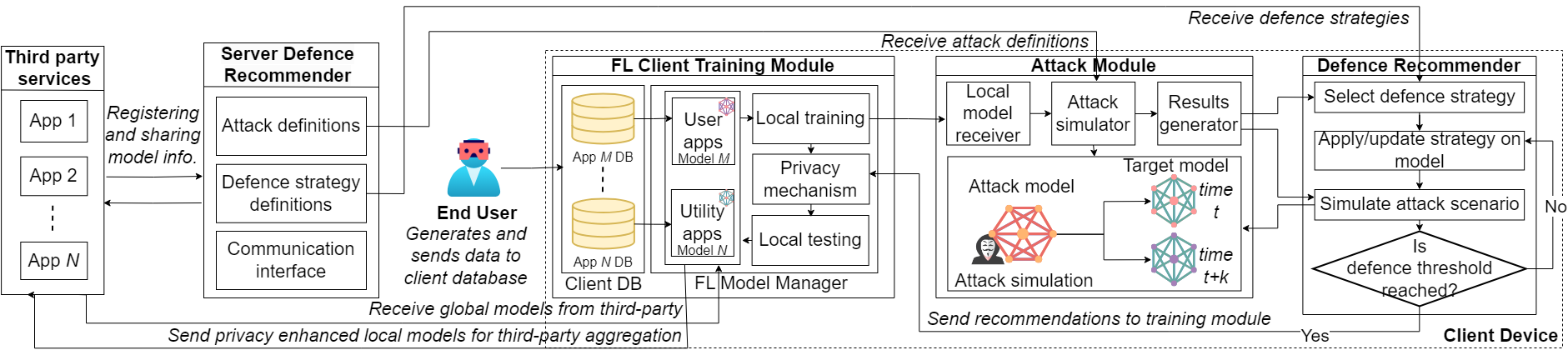
Rec-Def: A Recommendation-based Defence Mechanism for Privacy Preservation in Federated Learning Systems
- Post by: Chamara Sandeepa, Bartlomiej Siniarski, Shen Wang and Madhusanka Liyanage
- November 19, 2023
- Comments off
An emergence of attention and regulations on consumer privacy can be observed over the recent years with the ubiquitous availability of IoT systems handling personal data. Federated Learning (FL) arises as a privacy-preserved Machine Learning (ML) technique where data can be kept private within these devices without transmitting to third parties. Yet, many privacy attacks against FL can still leak private and sensitive information. Though solutions are currently available, they may not fit into the scope of IoT devices and may lead to sub-optimal results for the FL process. To balance these trade-offs, we propose a consumer-first approach to privacy protection where local privacy preservation is done via a privacy recommendation system. To evaluate the level of vulnerability of the local FL models, we use existing attacks and propose a novel time-based inference attack to test the resilience of FL models. Based on the vulnerability assessment, privacy recommendations are applied to local FL models based on a new gradient-split mechanism that adds a perturbation mask to the updates. Our experiments demonstrate the attacks can be mitigated effectively via the proposed mechanisms, with enhanced privacy and minimum compromise to the FL model utility.