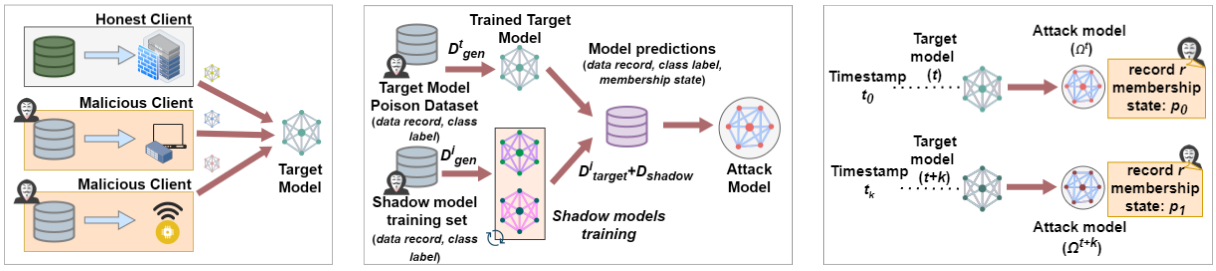
FL-TIA: Novel Time Inference Attacks on Federated Learning
- Post by: Chamara Sandeepa, Bartlomiej Siniarski, Shen Wang and Madhusanka Liyanage
- November 19, 2023
- Comments off
Federated Learning (FL) is an emerging privacy-preserved distributed Machine Learning (ML) technique where multiple clients can contribute to training an ML model without sharing private data. Even though FL offers a certain level of privacy by design, recent works show that FL is vulnerable to numerous privacy attacks. One of the key features of FL is the continuous training of FL models over many cycles through time. Observing changes in FL models over time can lead to inferring information on changes to private and sensitive data used in the FL process. However, this potential leakage of private information is not yet investigated significantly. Therefore, this paper introduces a new form of inference-based privacy attacks called FL Time Inference Attacks (FL-TIA). These attacks can reveal private time-related properties such as the presence or absence of a sensitive feature over time and if it is periodical. We consider two forms of such FL-TIA: i.e. identifying changes in membership of target data records over training rounds and detecting significant events in clients over time by observing differences in FL models. We use the network Intrusion Detection System (IDS) as a use case to demonstrate the impact of our attack. We propose a continuous updating attack model method for membership variation detection by sustaining the accuracy of the attack. Furthermore, we provide an efficient detection method that can identify model changes using cosine similarity metric and one-shot mapping on shadow model training.