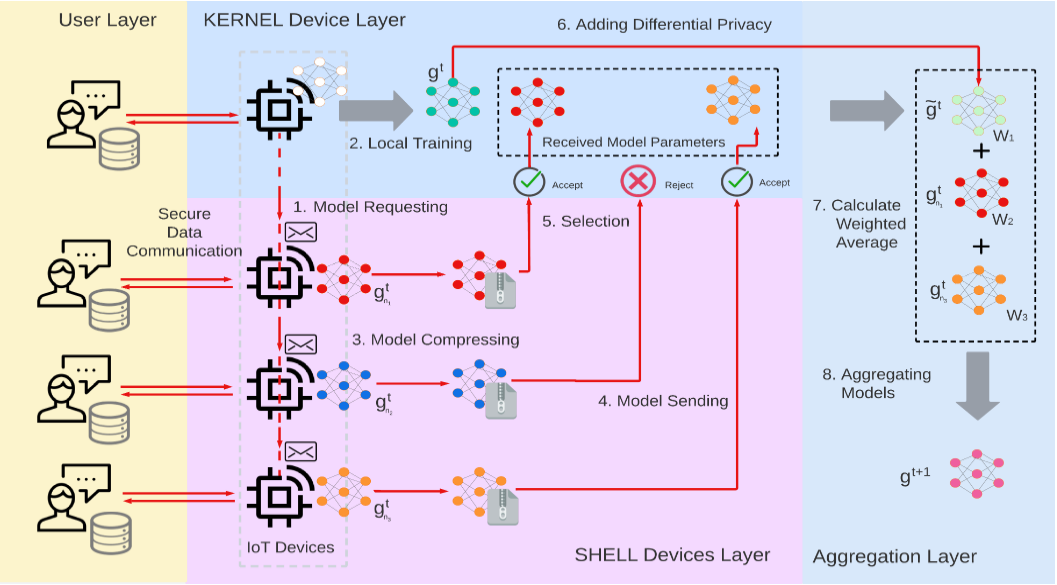
Privacy-preserved Collaborative Federated Learning Platform for Industrial Internet of Things
- Post by: Lakshan Pathiraja, Isuru Lakshan, Kavini Kushani, Chamara Sandeepa, Tharindu Gamage, Thilina Weerasinghe and Madhusanka Liyanage
- November 19, 2023
- Comments off
Federated learning (FL) is an intriguing approach to privacy-preserving collaborative learning. Decentralised FL is achieving increased favour for investigation due to the mitigation of vulnerability for a single point of failure and more controllability for end users over their models. However, many existing decentralised FL systems face limitations, such as privacy concerns, latency in aggregation, and real-world implementation challenges. To mitigate these issues, we introduce a novel FL protocol with a decentralised Peer-to-Peer (P2P) system using Differential Privacy (DP). It consists of a decentralised accuracy-based weighted averaging mechanism for both enhanced privacy and model aggregating accuracy. We implement our system in both virtual and real environments to evaluate the performance of the proposed mechanism. Moreover, we perform a comparative analysis of our proposal with both existing centralised and decentralised systems. To practically demonstrate the work, we consider a real-world use case of a recommendation system using smart carts. Experimental results show that our novel approach efficiently performs privacy-preserved aggregations over a decentralised peer network.