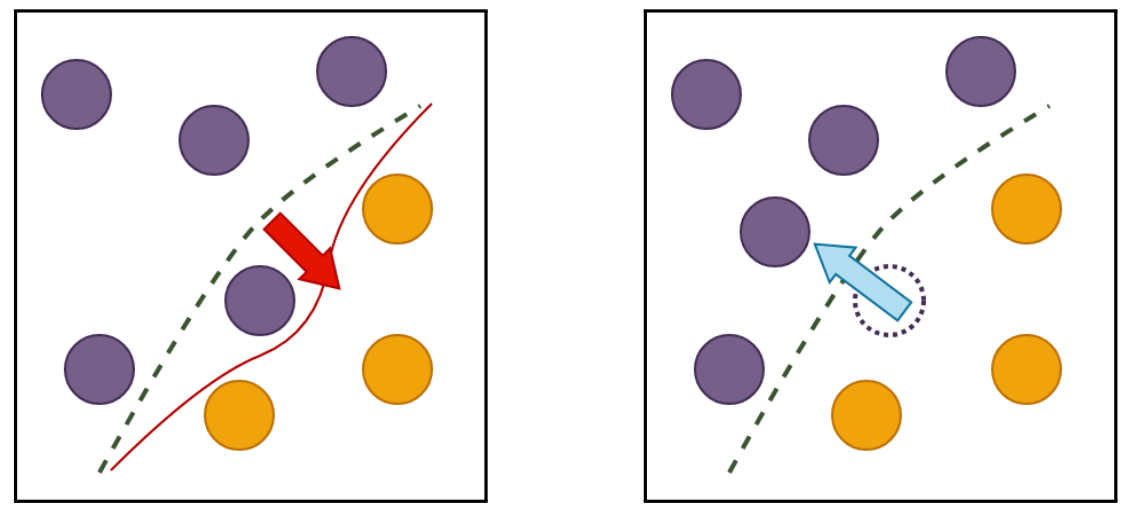
SHERPA: Explainable Robust Algorithms for Privacy-Preserved Federated Learning in Future Networks to Defend Against Data Poisoning Attacks
- Post by: Chamara Sandeepa, Bartlomiej Siniarski, Shen Wang and Madhusanka Liyanage
- February 10, 2025
- No Comment
With the rapid progression of communication and localisation of big data over billions of devices, distributed Machine Learning (ML) techniques are emerging to cater for the development of Artificial Intelligence (AI)-based services in a distributed manner. Federated Learning (FL) is such an innovative approach to achieve a privacy-preserved AI that facilitates ML model sharing and aggregation while keeping the participants’ data at the original source. However, recent research has investigated threats from poisoning attacks in FL. Several robust algorithms based on techniques such as similarity metrics or anomaly filtering are proposed as solutions. Yet, these approaches do not focus on investigating the intentions of the attackers or providing justifications and evidence for suspecting the behaviour of clients who are considered poisoners. Therefore, we propose SHERPA, a robust algorithm that uses Shapley Additive Explanations (SHAP) to identify potential poisoners in an FL system. We investigate how SHAP feature attributions can provide information on poisoners since the attributions depict the overall behaviour of the models by observing if the model prioritises relevant features in a given class when providing predictions. Based on this, we develop a novel algorithm to differentiate poisoners via feature attribution clustering. We launch data poisoning attacks for different scenarios on multiple datasets and showcase our solution to mitigate the attacks. Furthermore, we show that privacy-targeted poisoning attacks can be mitigated with our approach. Accompanying the Explainable AI (XAI) technique for defence, our study reveals the potential for post-hoc feature attributions in countering data poisoning attacks with better explainability and improved justification in eliminating potentially malicious clients in the aggregation process.