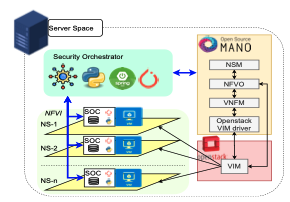
A Federated Learning Approach for Improving Security in Network Slicing
- Post by: Shalitha Wijethilaka and Madhusanka Liyanage
- November 17, 2023
- Comments off
Network Slicing (NS) is a predominant technology in future telecommunication networks, including Fifth Generation (5G), which supports the realization of heterogeneous applications and services. It allows the allocation of a dedicated logical network slice of the physical network to each application. Security is one of the paramount challenges in an NS ecosystem. Several technologies, including Machine Learning (ML), have been proposed to mitigate security challenges in 5G networks. However, the use of ML for NS security is not properly implemented. Especially, the scarcity of coordination and the difficulties of privacy-protected information sharing between slices cause failures and performance degradation of these ML based NS security solutions. To address this issue, this paper proposes a novel Federated Learning (FL) based coordinated security orchestration architecture named Federated Learning enabled Security Orchestrator (FLeSO) to centrally perform security operations in a slicing ecosystem while preserving the privacy of the data. In addition, the proposed FLeSO architecture enables features such as proactive security deployment and steady security level maintenance independent of the slicing strategy. The proposed architecture is implemented in a real-world slicing testbed, and a comprehensive set of experiments are performed to evaluate the effectiveness of the proposed FLeSO architecture. The test results illustrate the significant advantage of the proposed approach over the legacy system in terms of improving the security of an NS ecosystem.