Large Language Models (LLMs) have revolutionized natural language processing, but deploying them in resource-constrained environments and privacy-sensitive domains remains challenging. This paper introduces the Distributed Large Language Model (DisLLM), a novel distributed learning framework that addresses privacy preservation and computational efficiency issues in LLM fine-tuning and inference. DisLLM leverages the Splitfed Learning (SFL) approach, combining […]
Read More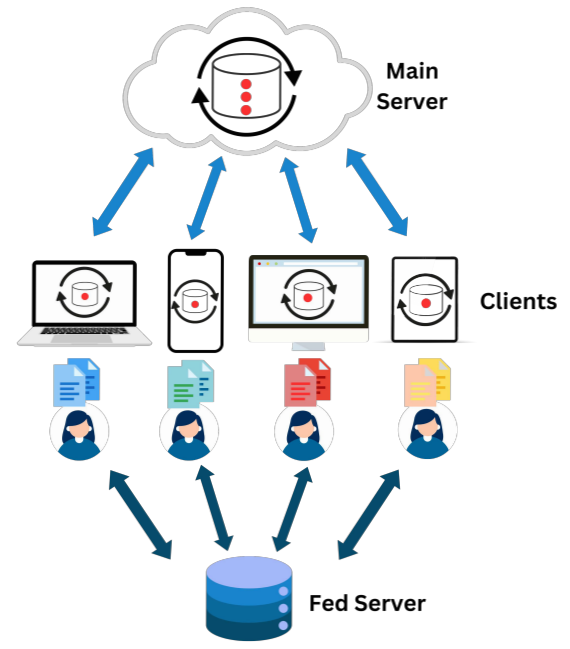